
NEW YORK - Researchers led by an Indian-origin scientist have developed a software that can turn any smartphone into an eye-tracking device, a discovery that can help in psychological experiments and marketing research. In addition to making existing applications of eye-tracking technology more accessible, the system could enable new computer interfaces or help detect signs of incipient neurological disease or mental illness.
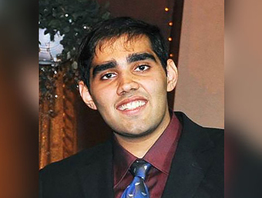
Since few people have the external devices, there's no big incentive to develop applications for them. “Since there are no applications, there's no incentive for people to buy the devices. We thought we should break this circle and try to make an eye tracker that works on a single mobile device, using just your front-facing camera,” explained Aditya Khosla, graduate student in electrical engineering and computer science at Massachusetts Institute of Technology (MIT). Khosla and his colleagues from MIT and University of Georgia built their eye tracker using machine learning, a technique in which computers learn to perform tasks by looking for patterns in large sets of training examples.
Currently, Khosla says, their training set includes examples of gaze patterns from 1,500 mobile-device users.
Previously, the largest data sets used to train experimental eye-tracking systems had topped out at about 50 users.
To assemble data sets, "most other groups tend to call people into the lab," Khosla says. "It's really hard to scale that up. Calling 50 people in itself is already a fairly tedious process. But we realised we could do this through crowdsourcing,” he added.
In the paper, the researchers report an initial round of experiments, using training data drawn from 800 mobile-device users.
On that basis, they were able to get the system's margin of error down to 1.5 centimetres, a twofold improvement over previous experimental systems. The researchers recruited application users through Amazon's Mechanical Turk crowdsourcing site and paid them a small fee for each successfully executed tap. The data set contains, on average, 1,600 images for each user.
The team from MIT's Computer Science and Artificial Intelligence Laboratory and the University of Georgia described their new system in a paper set to presented at the "Computer Vision and Pattern Recognition" conference in Las Vegas on June 28.
Currently, Khosla says, their training set includes examples of gaze patterns from 1,500 mobile-device users.
Previously, the largest data sets used to train experimental eye-tracking systems had topped out at about 50 users.
To assemble data sets, "most other groups tend to call people into the lab," Khosla says. "It's really hard to scale that up. Calling 50 people in itself is already a fairly tedious process. But we realised we could do this through crowdsourcing,” he added.
In the paper, the researchers report an initial round of experiments, using training data drawn from 800 mobile-device users.
On that basis, they were able to get the system's margin of error down to 1.5 centimetres, a twofold improvement over previous experimental systems. The researchers recruited application users through Amazon's Mechanical Turk crowdsourcing site and paid them a small fee for each successfully executed tap. The data set contains, on average, 1,600 images for each user.
The team from MIT's Computer Science and Artificial Intelligence Laboratory and the University of Georgia described their new system in a paper set to presented at the "Computer Vision and Pattern Recognition" conference in Las Vegas on June 28.